Large language models (LLMs) have significantly transformed natural language processing (NLP) and artificial intelligence (AI). Models like GPT-4 and BERT demonstrate remarkable capabilities in understanding and generating human-like text. However, the challenges and applications of large language models present several issues that need to be addressed for their effective utilization. Businesses and researchers are rapidly adopting LLMs to enhance various applications, from customer service chatbots to advanced scientific research.
Transformative Applications of Large Language Models
Large language models have become integral to various fields, showcasing their versatility and power.
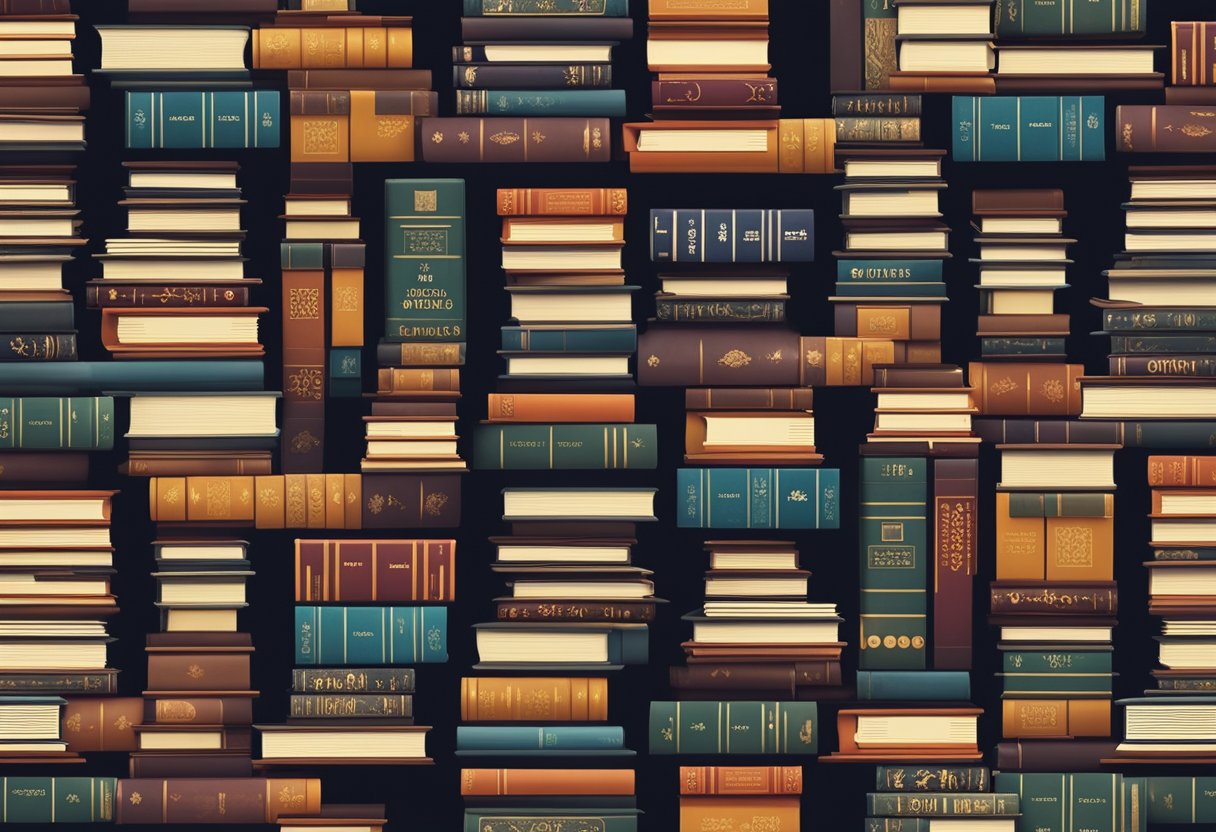
Practical Applications and Use Cases
- Customer Service and Virtual Assistants: LLMs are at the heart of many customer service solutions, such as chatbots and virtual assistants like Siri and Alexa. These models help streamline operations, handle inquiries, and perform question-answering tasks, reducing the need for manual intervention.
- Content Creation and Personalization: LLMs are revolutionizing content creation, aiding writers by generating ideas, drafting articles, and even providing personalized tutoring systems. They support creative industries by automating writing and enhancing productivity through effective text-based communication.
- Scientific Research: In life sciences, LLMs are used to analyze and interpret vast amounts of data. They assist in predicting protein structures, understanding genetic codes, and accelerating drug discovery processes, highlighting their potential to address complex biological challenges.
Large Language Models in Life Sciences
In the realm of life sciences, scientists use LLMs to analyze and interpret vast amounts of data efficiently. These models assist in predicting protein structures, understanding genetic codes, and accelerating drug discovery processes. Such breakthroughs illuminate the incredible potential of LLMs in revolutionizing how we address complex biological challenges.
- Data Analysis and Interpretation: Researchers use LLMs for natural language understanding of scientific literature, enabling efficient information retrieval. Models like Copernicus AI apply LLM capabilities in genomic research and drug discovery, interpreting complex biological data to uncover patterns and insights that would otherwise require extensive manual effort.
- Enhanced Research Efficiency: By speeding up research processes, LLMs provide systematic surveys for better scientific understanding, thereby transforming the pace and quality of scientific discoveries.
Challenges of Large Language Models
Despite their transformative potential, LLMs present several challenges that need to be addressed to ensure their effective and ethical use.
Technical Challenges of LLMs
- Training Data and Computational Resources: Training LLMs requires vast datasets and sophisticated strategies. This involves extensive computational resources, making it difficult for smaller organizations to develop and deploy such models. Managing and curating high-quality datasets is also a significant challenge.
- Model Interpretability: Understanding how LLMs make decisions remains a complex issue. The opacity of these models can lead to difficulties in diagnosing errors and biases, making it crucial to develop methods for improving their interpretability and transparency.
- Scalability and Efficiency: As models scale up, maintaining efficiency and managing computational costs become increasingly challenging. Innovations in training strategies, like transfer learning and pruning, are essential to enhance model performance and efficiency without compromising scalability.
Ethical and Societal Challenges of LLMs
- Bias and Fairness: LLMs can inadvertently perpetuate biases present in the training data, leading to unfair or harmful outcomes. Addressing bias and ensuring fairness in AI systems are critical for their ethical deployment.
- Data Privacy: The use of large datasets raises concerns about data privacy and security. Ensuring that LLMs comply with data protection regulations and respect user privacy is paramount.
- Impact on Employment: While LLMs improve service efficiency and enable new technologies, they may also lead to job displacement in certain sectors. Balancing technological advancement with social responsibility is necessary to mitigate negative impacts on the workforce.
Advances in Large Language Model Technology
The ongoing advancements in LLM technology continue to expand their capabilities and applications.
Architectural Innovations
- Transformers and Attention Mechanisms: LLM architecture heavily relies on the Transformer model and the attention mechanism. These innovations allow models to focus on different parts of a sentence dynamically, enhancing their understanding and processing of natural language.
- Foundation Models: Recent developments have introduced foundation models like GPT-4, which can handle diverse applications with minimal additional training. These models support a wide range of machine learning tasks, from text generation to complex NLP tasks, highlighting their versatility.
Emerging Technologies
- Artificial General Intelligence (AGI): Integrating LLMs into AGI research aims to create systems with more general problem-solving capabilities. While still in its early stages, this research holds the potential to develop AI systems that can perform a broader array of tasks with human-like understanding.
- Near-Field Scanning Optical Microscopy (NSOM) and Atomic Force Microscopy (AFM): Although not directly related to LLMs, these advanced microscopy techniques benefit from AI and machine learning advancements, showcasing the interdisciplinary impact of LLM technology.
Ethical Considerations and Responsible AI Usage
Ensuring the ethical deployment of LLMs is crucial to addressing the challenges and applications of large language models, maximizing their benefits while minimizing potential harms.
Data Privacy and Security
- Regulatory Compliance: Adhering to data protection regulations and ensuring that LLMs respect user privacy are essential for maintaining trust and reliability in AI systems.
- Transparency and Accountability: Developing methods to improve the interpretability of LLMs can enhance transparency and accountability, making it easier to diagnose errors and biases.
Social Responsibility
- Bias Mitigation: Addressing biases in LLMs involves continuous monitoring and updating of training data to ensure fairness and avoid harmful outcomes.
- Workforce Impact: Balancing technological advancement with social responsibility requires strategies to mitigate job displacement, such as reskilling programs and supportive policies for affected workers.
Large language models are transforming various fields with their remarkable capabilities in understanding and generating human-like text. However, addressing the challenges and applications of large language models, including technical, ethical, and societal issues, is essential for their sustainable and responsible use. By leveraging LLMs thoughtfully, we can harness their potential to drive innovation and improve efficiency across diverse applications.